VAX: Using Existing Video and Audio-based Activity Recognition Models to Bootstrap Privacy-Sensitive Sensors
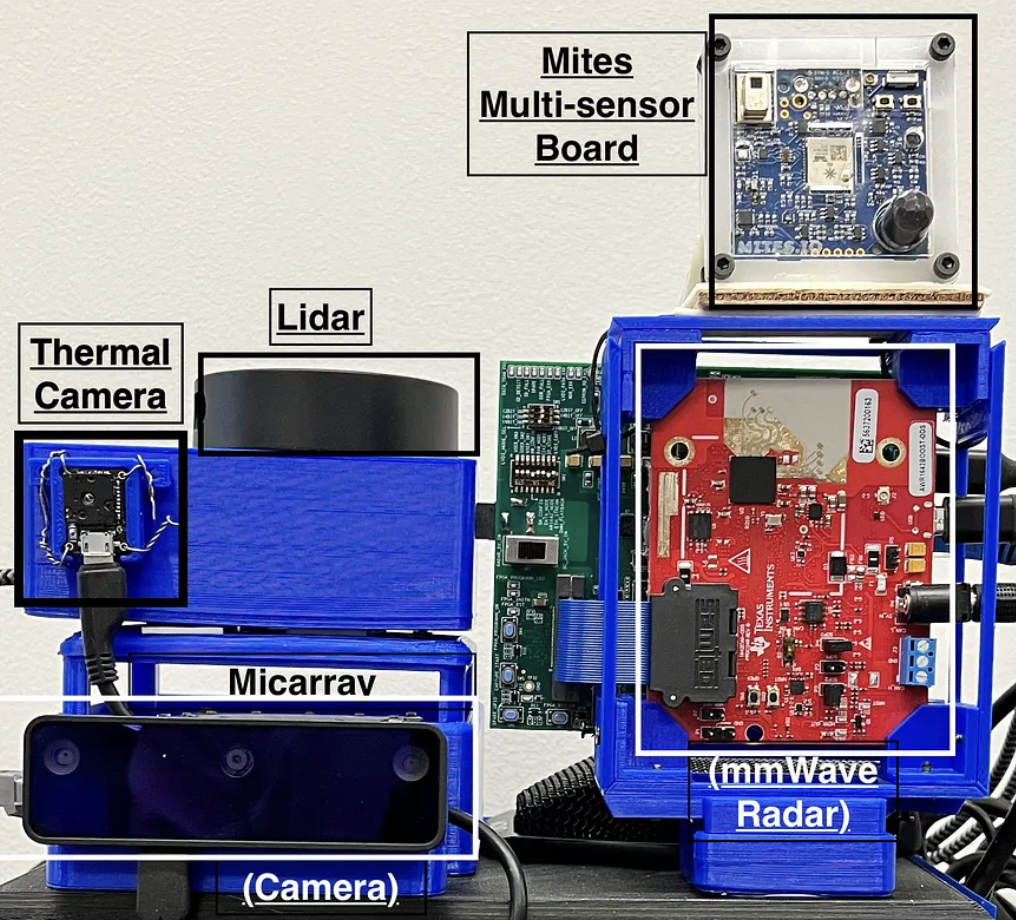
The use of audio and video modalities for Human Activity Recognition (HAR) is common, given the richness of the data and the availability of pre-trained ML models using a large corpus of labeled training data. However, audio and video sensors also lead to significant consumer privacy concerns. A key limitation of prior approaches is that most of them do not readily generalize across environments and require significant in-situ training data. In this paper, we generalize this concept to create a novel system called VAX (Video/Audio to ‘X’), where training labels acquired from existing Video/Audio ML models are used to train ML models for a wide range of ‘X’ privacy-sensitive sensors. Once the ML models for the privacy-sensitive sensors are trained, with little to no user involvement, the Audio/Video sensors can be removed altogether to protect the user’s privacy better. We built and deployed VAX in ten participants’ homes while they performed 17 common activities of daily living.